BBH模型和态密度分布参考这篇:BBH高阶拓扑绝缘体模型(附Python代码)。BBH模型和nested Wilson loop公式见参考文献[1-3]。本篇计算的nested Wilson loop仅仅是考虑能带非简并的情况。
一、能带
先画个能带。为了避免重复写代码,可以调用开源软件包Guan中的函数( https://py.guanjihuan.com)。
Python代码:
"""
This code is supported by the website: https://www.guanjihuan.com
The newest version of this code is on the web page: https://www.guanjihuan.com/archives/17984
"""
import numpy as np
import cmath
from math import *
import functools
def hamiltonian(kx, ky): # BBH model
# label of atoms in a unit cell
# (2) —— (0)
# | |
# (1) —— (3)
gamma_x = 0.5 # hopping inside one unit cell
lambda_x = 1 # hopping between unit cells
gamma_y = gamma_x
lambda_y = lambda_x
h = np.zeros((4, 4), dtype=complex)
h[0, 2] = gamma_x+lambda_x*cmath.exp(1j*kx)
h[1, 3] = gamma_x+lambda_x*cmath.exp(-1j*kx)
h[0, 3] = gamma_y+lambda_y*cmath.exp(1j*ky)
h[1, 2] = -gamma_y-lambda_y*cmath.exp(-1j*ky)
h[2, 0] = np.conj(h[0, 2])
h[3, 1] = np.conj(h[1, 3])
h[3, 0] = np.conj(h[0, 3])
h[2, 1] = np.conj(h[1, 2])
return h
def main():
kx = np.arange(-pi, pi, 0.05)
ky = np.arange(-pi, pi, 0.05)
eigenvalue_array = calculate_eigenvalue_with_two_parameters(kx, ky, hamiltonian)
plot_3d_surface(kx, ky, eigenvalue_array, xlabel='kx', ylabel='ky', zlabel='E', title='BBH bands')
hamiltonian0 = functools.partial(hamiltonian, ky=0)
eigenvalue_array = calculate_eigenvalue_with_one_parameter(kx, hamiltonian0)
plot(kx, eigenvalue_array, xlabel='kx', ylabel='E', title='BBH bands ky=0')
# import guan
# eigenvalue_array = guan.calculate_eigenvalue_with_two_parameters(kx, ky, hamiltonian)
# guan.plot_3d_surface(kx, ky, eigenvalue_array, xlabel='kx', ylabel='ky', zlabel='E', title='BBH bands')
# hamiltonian0 = functools.partial(hamiltonian, ky=0)
# eigenvalue_array = guan.calculate_eigenvalue_with_one_parameter(kx, hamiltonian0)
# guan.plot(kx, eigenvalue_array, xlabel='kx', ylabel='E', title='BBH bands ky=0')
def calculate_eigenvalue_with_one_parameter(x_array, hamiltonian_function, print_show=0):
dim_x = np.array(x_array).shape[0]
i0 = 0
if np.array(hamiltonian_function(0)).shape==():
eigenvalue_array = np.zeros((dim_x, 1))
for x0 in x_array:
hamiltonian = hamiltonian_function(x0)
eigenvalue_array[i0, 0] = np.real(hamiltonian)
i0 += 1
else:
dim = np.array(hamiltonian_function(0)).shape[0]
eigenvalue_array = np.zeros((dim_x, dim))
for x0 in x_array:
if print_show==1:
print(x0)
hamiltonian = hamiltonian_function(x0)
eigenvalue, eigenvector = np.linalg.eigh(hamiltonian)
eigenvalue_array[i0, :] = eigenvalue
i0 += 1
return eigenvalue_array
def calculate_eigenvalue_with_two_parameters(x_array, y_array, hamiltonian_function, print_show=0, print_show_more=0):
dim_x = np.array(x_array).shape[0]
dim_y = np.array(y_array).shape[0]
if np.array(hamiltonian_function(0,0)).shape==():
eigenvalue_array = np.zeros((dim_y, dim_x, 1))
i0 = 0
for y0 in y_array:
j0 = 0
for x0 in x_array:
hamiltonian = hamiltonian_function(x0, y0)
eigenvalue_array[i0, j0, 0] = np.real(hamiltonian)
j0 += 1
i0 += 1
else:
dim = np.array(hamiltonian_function(0, 0)).shape[0]
eigenvalue_array = np.zeros((dim_y, dim_x, dim))
i0 = 0
for y0 in y_array:
j0 = 0
if print_show==1:
print(y0)
for x0 in x_array:
if print_show_more==1:
print(x0)
hamiltonian = hamiltonian_function(x0, y0)
eigenvalue, eigenvector = np.linalg.eigh(hamiltonian)
eigenvalue_array[i0, j0, :] = eigenvalue
j0 += 1
i0 += 1
return eigenvalue_array
def plot(x_array, y_array, xlabel='x', ylabel='y', title='', fontsize=20, labelsize=20, show=1, save=0, filename='a', file_format='.jpg', dpi=300, style='', y_min=None, y_max=None, linewidth=None, markersize=None, adjust_bottom=0.2, adjust_left=0.2):
import matplotlib.pyplot as plt
fig, ax = plt.subplots()
plt.subplots_adjust(bottom=adjust_bottom, left=adjust_left)
ax.plot(x_array, y_array, style, linewidth=linewidth, markersize=markersize)
ax.grid()
ax.set_title(title, fontsize=fontsize, fontfamily='Times New Roman')
ax.set_xlabel(xlabel, fontsize=fontsize, fontfamily='Times New Roman')
ax.set_ylabel(ylabel, fontsize=fontsize, fontfamily='Times New Roman')
if y_min!=None or y_max!=None:
if y_min==None:
y_min=min(y_array)
if y_max==None:
y_max=max(y_array)
ax.set_ylim(y_min, y_max)
ax.tick_params(labelsize=labelsize)
labels = ax.get_xticklabels() + ax.get_yticklabels()
[label.set_fontname('Times New Roman') for label in labels]
if save == 1:
plt.savefig(filename+file_format, dpi=dpi)
if show == 1:
plt.show()
plt.close('all')
def plot_3d_surface(x_array, y_array, matrix, xlabel='x', ylabel='y', zlabel='z', title='', fontsize=20, labelsize=15, show=1, save=0, filename='a', file_format='.jpg', dpi=300, z_min=None, z_max=None, rcount=100, ccount=100):
import matplotlib.pyplot as plt
from matplotlib import cm
from matplotlib.ticker import LinearLocator
matrix = np.array(matrix)
fig, ax = plt.subplots(subplot_kw={"projection": "3d"})
plt.subplots_adjust(bottom=0.1, right=0.65)
x_array, y_array = np.meshgrid(x_array, y_array)
if len(matrix.shape) == 2:
surf = ax.plot_surface(x_array, y_array, matrix, rcount=rcount, ccount=ccount, cmap=cm.coolwarm, linewidth=0, antialiased=False)
elif len(matrix.shape) == 3:
for i0 in range(matrix.shape[2]):
surf = ax.plot_surface(x_array, y_array, matrix[:,:,i0], rcount=rcount, ccount=ccount, cmap=cm.coolwarm, linewidth=0, antialiased=False)
ax.set_title(title, fontsize=fontsize, fontfamily='Times New Roman')
ax.set_xlabel(xlabel, fontsize=fontsize, fontfamily='Times New Roman')
ax.set_ylabel(ylabel, fontsize=fontsize, fontfamily='Times New Roman')
ax.set_zlabel(zlabel, fontsize=fontsize, fontfamily='Times New Roman')
ax.zaxis.set_major_locator(LinearLocator(5))
ax.zaxis.set_major_formatter('{x:.2f}')
if z_min!=None or z_max!=None:
if z_min==None:
z_min=matrix.min()
if z_max==None:
z_max=matrix.max()
ax.set_zlim(z_min, z_max)
ax.tick_params(labelsize=labelsize)
labels = ax.get_xticklabels() + ax.get_yticklabels() + ax.get_zticklabels()
[label.set_fontname('Times New Roman') for label in labels]
cax = plt.axes([0.8, 0.1, 0.05, 0.8])
cbar = fig.colorbar(surf, cax=cax)
cbar.ax.tick_params(labelsize=labelsize)
for l in cbar.ax.yaxis.get_ticklabels():
l.set_family('Times New Roman')
if save == 1:
plt.savefig(filename+file_format, dpi=dpi)
if show == 1:
plt.show()
plt.close('all')
if __name__ == '__main__':
main()
运行结果:
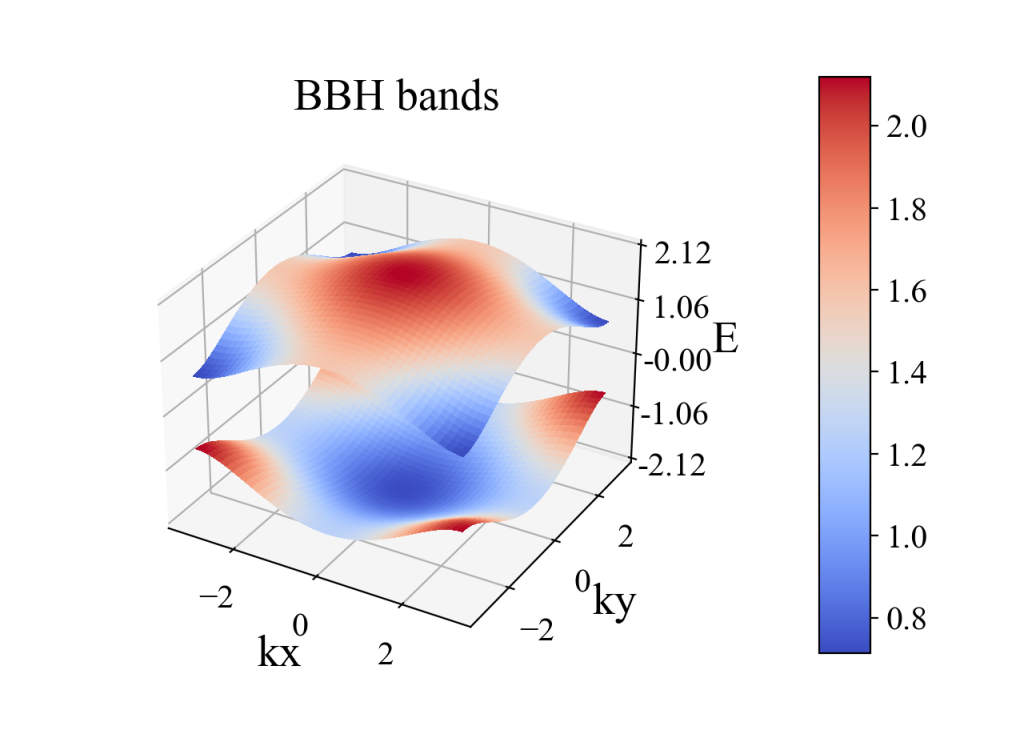
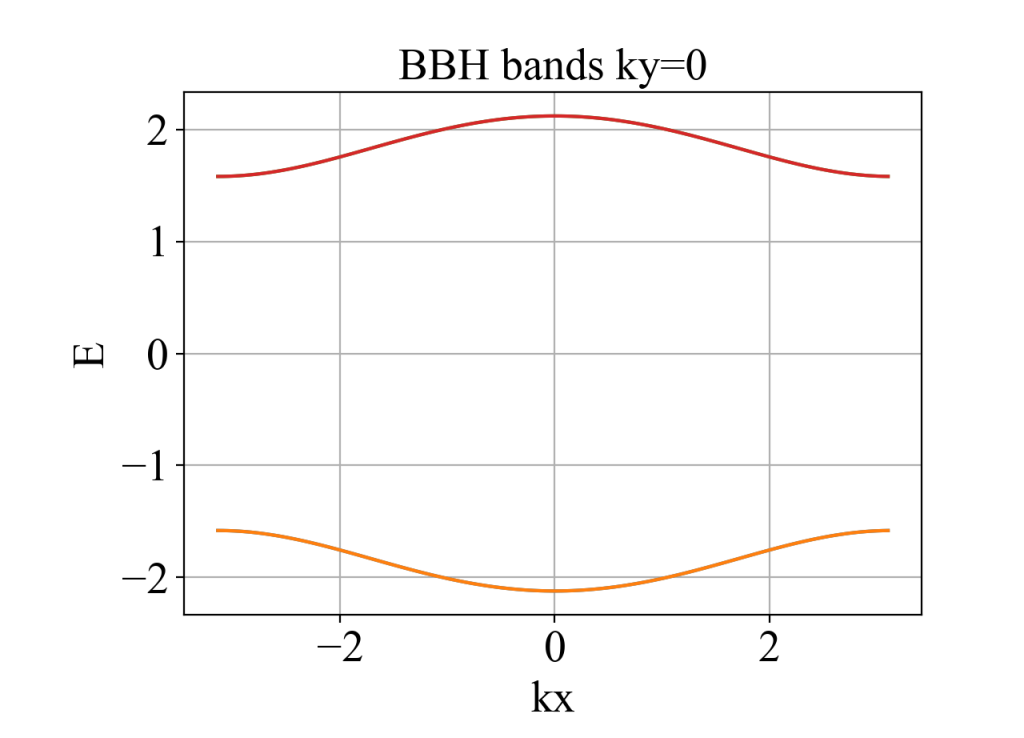
二、Wilson loop
Python代码:
"""
This code is supported by the website: https://www.guanjihuan.com
The newest version of this code is on the web page: https://www.guanjihuan.com/archives/17984
"""
import numpy as np
import cmath
from math import *
def hamiltonian(kx, ky): # BBH model
# label of atoms in a unit cell
# (2) —— (0)
# | |
# (1) —— (3)
gamma_x = 0.5 # hopping inside one unit cell
lambda_x = 1 # hopping between unit cells
gamma_y = gamma_x
lambda_y = lambda_x
h = np.zeros((4, 4), dtype=complex)
h[0, 2] = gamma_x+lambda_x*cmath.exp(1j*kx)
h[1, 3] = gamma_x+lambda_x*cmath.exp(-1j*kx)
h[0, 3] = gamma_y+lambda_y*cmath.exp(1j*ky)
h[1, 2] = -gamma_y-lambda_y*cmath.exp(-1j*ky)
h[2, 0] = np.conj(h[0, 2])
h[3, 1] = np.conj(h[1, 3])
h[3, 0] = np.conj(h[0, 3])
h[2, 1] = np.conj(h[1, 2])
return h
def main():
Num_kx = 100
Num_ky = 100
kx_array = np.linspace(-pi, pi, Num_kx)
ky_array = np.linspace(-pi, pi, Num_ky)
nu_x_array = []
for ky in ky_array:
vector1_array = []
vector2_array = []
for kx in kx_array:
eigenvalue, eigenvector = np.linalg.eigh(hamiltonian(kx, ky))
if kx != pi:
vector1_array.append(eigenvector[:, 0])
vector2_array.append(eigenvector[:, 1])
else:
# 这里是为了-pi和pi有相同的波函数,使得Wilson loop的值与波函数规范无关。
vector1_array.append(vector1_array[0])
vector2_array.append(vector2_array[0])
W_x_k = np.eye(2, dtype=complex)
for i0 in range(Num_kx-1):
F = np.zeros((2, 2), dtype=complex)
F[0, 0] = np.dot(vector1_array[i0+1].transpose().conj(), vector1_array[i0])
F[1, 1] = np.dot(vector2_array[i0+1].transpose().conj(), vector2_array[i0])
F[0, 1] = np.dot(vector1_array[i0+1].transpose().conj(), vector2_array[i0])
F[1, 0] = np.dot(vector2_array[i0+1].transpose().conj(), vector1_array[i0])
W_x_k = np.dot(F, W_x_k)
eigenvalue, eigenvector = np.linalg.eig(W_x_k)
nu_x = np.log(eigenvalue)/2/pi/1j
for i0 in range(2):
if np.real(nu_x[i0]) < 0:
nu_x[i0] += 1
nu_x = np.sort(nu_x)
nu_x_array.append(nu_x.real)
plot(ky_array, nu_x_array, xlabel='ky', ylabel='nu_x', style='-', y_min=0, y_max=1)
# import guan
# guan.plot(ky_array, nu_x_array, xlabel='ky', ylabel='nu_x', style='-', y_min=0, y_max=1)
def plot(x_array, y_array, xlabel='x', ylabel='y', title='', fontsize=20, labelsize=20, show=1, save=0, filename='a', file_format='.jpg', dpi=300, style='', y_min=None, y_max=None, linewidth=None, markersize=None, adjust_bottom=0.2, adjust_left=0.2):
import matplotlib.pyplot as plt
fig, ax = plt.subplots()
plt.subplots_adjust(bottom=adjust_bottom, left=adjust_left)
ax.plot(x_array, y_array, style, linewidth=linewidth, markersize=markersize)
ax.grid()
ax.set_title(title, fontsize=fontsize, fontfamily='Times New Roman')
ax.set_xlabel(xlabel, fontsize=fontsize, fontfamily='Times New Roman')
ax.set_ylabel(ylabel, fontsize=fontsize, fontfamily='Times New Roman')
if y_min!=None or y_max!=None:
if y_min==None:
y_min=min(y_array)
if y_max==None:
y_max=max(y_array)
ax.set_ylim(y_min, y_max)
ax.tick_params(labelsize=labelsize)
labels = ax.get_xticklabels() + ax.get_yticklabels()
[label.set_fontname('Times New Roman') for label in labels]
if save == 1:
plt.savefig(filename+file_format, dpi=dpi)
if show == 1:
plt.show()
plt.close('all')
if __name__ == '__main__':
main()
运行结果:
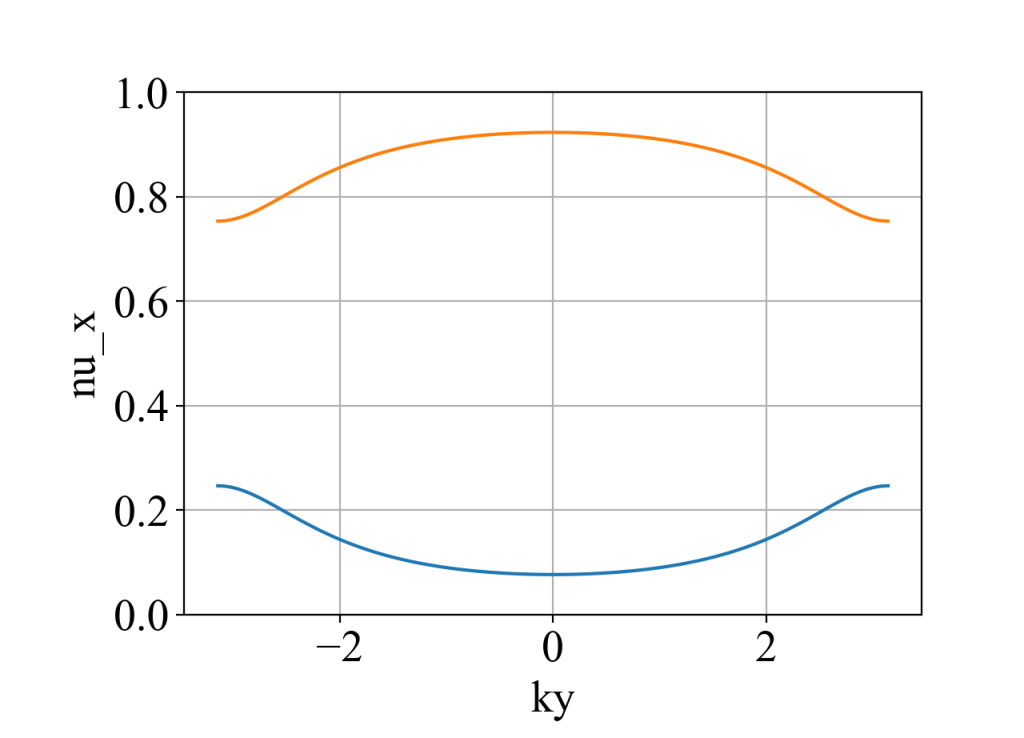
nu_y的计算类似。
三、nested Wilson loop
Python代码(非简并的情况):
"""
This code is supported by the website: https://www.guanjihuan.com
The newest version of this code is on the web page: https://www.guanjihuan.com/archives/17984
"""
import numpy as np
import cmath
from math import *
def hamiltonian(kx, ky): # BBH model
# label of atoms in a unit cell
# (2) —— (0)
# | |
# (1) —— (3)
gamma_x = 0.5 # hopping inside one unit cell
lambda_x = 1 # hopping between unit cells
gamma_y = gamma_x
lambda_y = lambda_x
x_symmetry_breaking_1 = 0.000000000000 # default (not breaking): zero
x_symmetry_breaking_2 = 1.0000000000001 # default (not breaking): unity
y_symmetry_breaking_1 = 0.000000000000 # default (not breaking): zero
y_symmetry_breaking_2 = 1.000000000000 # default (not breaking): unity
h = np.zeros((4, 4), dtype=complex)
h[0, 0] = x_symmetry_breaking_1
h[1, 1] = y_symmetry_breaking_1
h[2, 2] = y_symmetry_breaking_1
h[3, 3] = x_symmetry_breaking_1
h[0, 2] = (gamma_x+lambda_x*cmath.exp(1j*kx))*y_symmetry_breaking_2
h[1, 3] = gamma_x+lambda_x*cmath.exp(-1j*kx)
h[0, 3] = gamma_y+lambda_y*cmath.exp(1j*ky)
h[1, 2] = (-gamma_y-lambda_y*cmath.exp(-1j*ky))*x_symmetry_breaking_2
h[2, 0] = np.conj(h[0, 2])
h[3, 1] = np.conj(h[1, 3])
h[3, 0] = np.conj(h[0, 3])
h[2, 1] = np.conj(h[1, 2])
return h
def main():
Num_kx = 30 # for wilson loop and nested wilson loop
Num_ky = 30 # for wilson loop and nested wilson loop
Num_kx2 = 20 # plot precision
Num_ky2 = 20 # plot precision
kx_array = np.linspace(-pi, pi, Num_kx)
ky_array = np.linspace(-pi, pi, Num_ky)
kx2_array = np.linspace(-pi, pi, Num_kx2)
ky2_array = np.linspace(-pi, pi, Num_ky2)
# Part I: calculate p_y_for_nu_x
p_y_for_nu_x_array = []
for kx in kx2_array:
print('kx=', kx)
w_vector_for_nu1_array = []
vector1_array = []
vector2_array = []
i0 = -1
for ky in ky_array:
eigenvalue, eigenvector = np.linalg.eigh(hamiltonian(kx, ky))
if ky != pi:
vector1_array.append(eigenvector[:, 0])
vector2_array.append(eigenvector[:, 1])
else:
vector1_array.append(vector1_array[0])
vector2_array.append(vector2_array[0])
i0=0
for ky in ky_array:
if ky != pi:
nu_x_vector_1, nu_x_vector_2 = get_nu_x_vector(kx_array, ky)
# the Wannier band subspaces
w_vector_for_nu1 = vector1_array[i0]*nu_x_vector_1[0]+vector2_array[i0]*nu_x_vector_1[1]
w_vector_for_nu1_array.append(w_vector_for_nu1)
else:
w_vector_for_nu1_array.append(w_vector_for_nu1_array[0])
i0 +=1
W_y_k_for_nu_x = 1
for i0 in range(Num_ky-1):
F_for_nu_x = np.dot(w_vector_for_nu1_array[i0+1].transpose().conj(), w_vector_for_nu1_array[i0])
W_y_k_for_nu_x = F_for_nu_x*W_y_k_for_nu_x
p_y_for_nu_x = np.log(W_y_k_for_nu_x)/2/pi/1j
if np.real(p_y_for_nu_x) < 0:
p_y_for_nu_x += 1
p_y_for_nu_x_array.append(p_y_for_nu_x.real)
print('p_y_for_nu_x=', p_y_for_nu_x)
plot(kx2_array, p_y_for_nu_x_array, xlabel='kx', ylabel='p_y_for_nu_x', style='-o', y_min=0, y_max=1)
# import guan
# guan.plot(kx2_array, p_y_for_nu_x_array, xlabel='kx', ylabel='p_y_for_nu_x', style='-o', y_min=0, y_max=1)
# Part II: calculate p_x_for_nu_y
p_x_for_nu_y_array = []
for ky in ky2_array:
w_vector_for_nu1_array = []
vector1_array = []
vector2_array = []
for kx in kx_array:
eigenvalue, eigenvector = np.linalg.eigh(hamiltonian(kx, ky))
if kx != pi:
vector1_array.append(eigenvector[:, 0])
vector2_array.append(eigenvector[:, 1])
else:
vector1_array.append(vector1_array[0])
vector2_array.append(vector2_array[0])
i0 = 0
for kx in kx_array:
if kx != pi:
nu_y_vector_1, nu_y_vector_2 = get_nu_y_vector(kx, ky_array)
# the Wannier band subspaces
w_vector_for_nu1 = vector1_array[i0]*nu_y_vector_1[0]+vector2_array[i0]*nu_y_vector_1[1]
w_vector_for_nu1_array.append(w_vector_for_nu1)
else:
w_vector_for_nu1_array.append(w_vector_for_nu1_array[0])
i0 += 1
W_x_k_for_nu_y = 1
for i0 in range(Num_ky-1):
F_for_nu_y = np.dot(w_vector_for_nu1_array[i0+1].transpose().conj(), w_vector_for_nu1_array[i0])
W_x_k_for_nu_y = F_for_nu_y*W_x_k_for_nu_y
p_x_for_nu_y = np.log(W_x_k_for_nu_y)/2/pi/1j
if np.real(p_x_for_nu_y) < 0:
p_x_for_nu_y += 1
p_x_for_nu_y_array.append(p_x_for_nu_y.real)
print('p_x_for_nu_y=', p_x_for_nu_y)
# print(sum(p_x_for_nu_y_array)/len(p_x_for_nu_y_array))
plot(ky2_array, p_x_for_nu_y_array, xlabel='ky', ylabel='p_x_for_nu_y', style='-o', y_min=0, y_max=1)
# import guan
# guan.plot(ky2_array, p_x_for_nu_y_array, xlabel='ky', ylabel='p_x_for_nu_y', style='-o', y_min=0, y_max=1)
def get_nu_x_vector(kx_array, ky):
Num_kx = len(kx_array)
vector1_array = []
vector2_array = []
for kx in kx_array:
eigenvalue, eigenvector = np.linalg.eigh(hamiltonian(kx, ky))
if kx != pi:
vector1_array.append(eigenvector[:, 0])
vector2_array.append(eigenvector[:, 1])
else:
vector1_array.append(vector1_array[0])
vector2_array.append(vector2_array[0])
W_x_k = np.eye(2, dtype=complex)
for i0 in range(Num_kx-1):
F = np.zeros((2, 2), dtype=complex)
F[0, 0] = np.dot(vector1_array[i0+1].transpose().conj(), vector1_array[i0])
F[1, 1] = np.dot(vector2_array[i0+1].transpose().conj(), vector2_array[i0])
F[0, 1] = np.dot(vector1_array[i0+1].transpose().conj(), vector2_array[i0])
F[1, 0] = np.dot(vector2_array[i0+1].transpose().conj(), vector1_array[i0])
W_x_k = np.dot(F, W_x_k)
eigenvalue, eigenvector = np.linalg.eig(W_x_k)
nu_x = np.log(eigenvalue)/2/pi/1j
nu_x_vector_1 = eigenvector[:, np.argsort(np.real(nu_x))[0]]
nu_x_vector_2 = eigenvector[:, np.argsort(np.real(nu_x))[1]]
return nu_x_vector_1, nu_x_vector_2
def get_nu_y_vector(kx, ky_array):
Num_ky = len(ky_array)
vector1_array = []
vector2_array = []
for ky in ky_array:
eigenvalue, eigenvector = np.linalg.eigh(hamiltonian(kx, ky))
if ky != pi:
vector1_array.append(eigenvector[:, 0])
vector2_array.append(eigenvector[:, 1])
else:
vector1_array.append(vector1_array[0])
vector2_array.append(vector2_array[0])
W_y_k = np.eye(2, dtype=complex)
for i0 in range(Num_ky-1):
F = np.zeros((2, 2), dtype=complex)
F[0, 0] = np.dot(vector1_array[i0+1].transpose().conj(), vector1_array[i0])
F[1, 1] = np.dot(vector2_array[i0+1].transpose().conj(), vector2_array[i0])
F[0, 1] = np.dot(vector1_array[i0+1].transpose().conj(), vector2_array[i0])
F[1, 0] = np.dot(vector2_array[i0+1].transpose().conj(), vector1_array[i0])
W_y_k = np.dot(F, W_y_k)
eigenvalue, eigenvector = np.linalg.eig(W_y_k)
nu_y = np.log(eigenvalue)/2/pi/1j
nu_y_vector_1 = eigenvector[:, np.argsort(np.real(nu_y))[0]]
nu_y_vector_2 = eigenvector[:, np.argsort(np.real(nu_y))[1]]
return nu_y_vector_1, nu_y_vector_2
def plot(x_array, y_array, xlabel='x', ylabel='y', title='', fontsize=20, labelsize=20, show=1, save=0, filename='a', file_format='.jpg', dpi=300, style='', y_min=None, y_max=None, linewidth=None, markersize=None, adjust_bottom=0.2, adjust_left=0.2):
import matplotlib.pyplot as plt
fig, ax = plt.subplots()
plt.subplots_adjust(bottom=adjust_bottom, left=adjust_left)
ax.plot(x_array, y_array, style, linewidth=linewidth, markersize=markersize)
ax.grid()
ax.set_title(title, fontsize=fontsize, fontfamily='Times New Roman')
ax.set_xlabel(xlabel, fontsize=fontsize, fontfamily='Times New Roman')
ax.set_ylabel(ylabel, fontsize=fontsize, fontfamily='Times New Roman')
if y_min!=None or y_max!=None:
if y_min==None:
y_min=min(y_array)
if y_max==None:
y_max=max(y_array)
ax.set_ylim(y_min, y_max)
ax.tick_params(labelsize=labelsize)
labels = ax.get_xticklabels() + ax.get_yticklabels()
[label.set_fontname('Times New Roman') for label in labels]
if save == 1:
plt.savefig(filename+file_format, dpi=dpi)
if show == 1:
plt.show()
plt.close('all')
if __name__ == '__main__':
main()
运算结果:
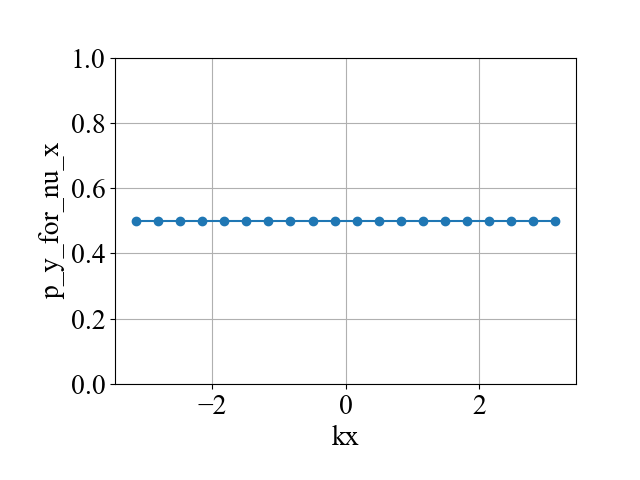
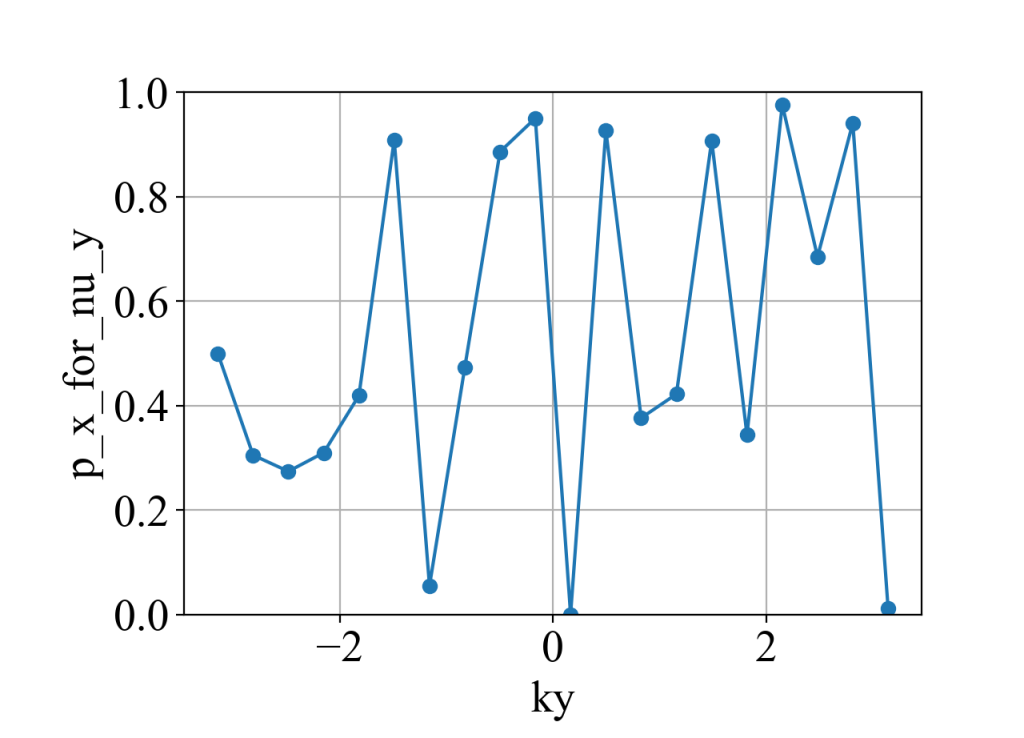
说明:
(1)代码中有些是对本征值做了重复计算,但为了代码直观就不做过多的优化。
(2)这里为了打开简并,打破了x方向的镜面对称性( x_symmetry_breaking_2 = 0.0000000000001 ),所以p_x_for_nu_y()没法保证是量子化。而由于y方向的镜面对称仍然保留,因此p_y_for_nu_y (
) 是量子化的,即[2]:
(3)以下的代码是处理能带简并时最简单情况,只做个波函数对调,仅做参考:
if kx == -pi:
vector1_array.append(eigenvector[:, 0])
vector2_array.append(eigenvector[:, 1])
elif kx != pi:
# 这里的判断是为了处理能带简并时最简单情况,只做个顺序对调。如果只是顺序反了,内积接近0。但实际会更复杂,不只是顺序的问题,而且任意的线性组合。
if np.abs(np.dot(vector1_array[-1].transpose().conj(), eigenvector[:, 0]))>0.5:
vector1_array.append(eigenvector[:, 0])
vector2_array.append(eigenvector[:, 1])
else:
vector1_array.append(eigenvector[:, 1])
vector2_array.append(eigenvector[:, 0])
else:
# 这里是为了-pi和pi有相同的波函数,使得Wilson loop的值与波函数规范无关。
vector1_array.append(vector1_array[0])
vector2_array.append(vector2_array[0])
(4)对于简并的情况,能带波函数可能需要旋转/幺正变换,得到固定的一个波函数基[3]。在这篇“非简并波函数和简并波函数的固定规范”中,给出了具体的代码。但用上这个固定规范后似乎也不能给出正确的结果,暂时还不知道怎么处理,暂且搁置了。
参考资料:
[1] Benalcazar et al., Quantized electric multipole insulators, science 357, 6346 (2017).
[4] 和YZZ同学的讨论
【说明:本站主要是个人的一些笔记和代码分享,内容可能会不定期修改。为了使全网显示的始终是最新版本,这里的文章未经同意请勿转载。引用请注明出处:https://www.guanjihuan.com】
老师,BBH模型的高对称点是多少,我想画一下沿着高对称点的能带图
路径应该和简单方格子的情况差不多的吧
好的,谢谢老师。
老师,在计算嵌套威尔逊环时,体系的哈密顿量为什么要这样写,为什么和计算能带和威尔逊环时写法不一样?另外,文章计算(Benalcazar, Bernevig, Hughes, Science 357,61–66 (2017))极化P_y_vx,公式显示对kx求和或者是积分,而您的代码中在哪里体现这个求和?
def hamiltonian(kx, ky): # BBH model
# label of atoms in a unit cell
# (2) —— (0)
# | |
# (1) —— (3)
gamma_x = 0.5 # hopping inside one unit cell
lambda_x = 1 # hopping between unit cells
gamma_y = gamma_x
lambda_y = lambda_x
x_symmetry_breaking_1 = 0.000000000000 # default (not breaking): zero
x_symmetry_breaking_2 = 1.0000000000001 # default (not breaking): unity
y_symmetry_breaking_1 = 0.000000000000 # default (not breaking): zero
y_symmetry_breaking_2 = 1.000000000000 # default (not breaking): unity
h = np.zeros((4, 4), dtype=complex)
h[0, 0] = x_symmetry_breaking_1
h[1, 1] = y_symmetry_breaking_1
h[2, 2] = y_symmetry_breaking_1
h[3, 3] = x_symmetry_breaking_1
h[0, 2] = (gamma_x+lambda_x*cmath.exp(1j*kx))*y_symmetry_breaking_2
h[1, 3] = gamma_x+lambda_x*cmath.exp(-1j*kx)
h[0, 3] = gamma_y+lambda_y*cmath.exp(1j*ky)
h[1, 2] = (-gamma_y-lambda_y*cmath.exp(-1j*ky))*x_symmetry_breaking_2
h[2, 0] = np.conj(h[0, 2])
h[3, 1] = np.conj(h[1, 3])
h[3, 0] = np.conj(h[0, 3])
h[2, 1] = np.conj(h[1, 2])
return h
因为这边我暂时无法处理能带简并的情况,所以通过微扰的方法打开能带简并。如果有其他方法可以计算,那么就不需要这么写。
log的加法体现在循环乘积:W_y_k_for_nu_x = F_for_nu_x*W_y_k_for_nu_x
作者您好,我想请问下这里最后说的:打破了x方向的镜面对称性,所以p_x_for_nu_y(p_{x}^{v_{y}^{\pm}})没法保证是量子化。而由于y方向的镜面对称仍然保留,因此p_y_for_nu_y (p_{y}^{v_{x}^{\pm}}) 是量子化的。
但是如果两个方向的镜面对称性都不破坏,为什么两个极化都不是量子化的呢?如果按照分析的话,应该p_x_for_nu_y和p_y_for_nu_y 两个都应该是量子化的,但是画图来看又不是?
如果两个方向的镜面对称性都不破坏,结果应该是量子化的。这里算的之所以没有量子化,是因为这时候能带存在简并,波函数不好区分处理,所以这时候数值计算结果是错误的。我暂时还不清楚怎么处理这个简并问题,这边只是算了下非简并的情况,所以要打破一些对称性。
哦哦哦好的感谢答主~
作者您好,请问一下,在一个正方形系统中,如果仅有两个角存在简并的角态,是否可以用nested wilson loop方法表征拓扑不变量
这个我不清楚,可以试着算下,看是否存在这个不变量。或者可能有新的拓扑不变量。
谢谢回复~
关老师,如果是6*6的矩阵,占据态的数目为3,在求解嵌套的wilson loop时,此时占据态波函数数目取1吗?
看得到的nu_x或nu_y中价带占据态有几个吧,可能是1,也可能是2,取决于多出的一条带在哪个位置,以及自己想要考虑什么样的问题。
谢谢回复
博主你好
你这个代码跟高阶拓扑的edge polarization是等价的么?
嗯,边界极化的量子化对应的就是高阶拓扑不变量
你这里可以计算实空间的 edge polarization 么?也就是说横坐标是 X 方向的格点数。
这里没有算。是指态密度分布吗?
指的是参考资料(1)中,图2的D图
嗯,我暂时没考虑。可以找对应的公式算。
关老师,我按照您这个Nested Wilson loop写了这个BBH模型在半开边界条件下的边界极化。按照公式的定义写出来但是算的不对。能麻烦您帮我看一下吗?算了快一周不知道哪里写的有问题。
clc; clear;
t=0.0; gamma_x=0.5; gamma_y=0.5; lambda=1; Ly=20; num_k=50; N_orb=4;%Ly 元胞数
N=num_k; N_occ=2; rho_j=zeros(Ly,1);
kx1=linspace(-pi,pi,num_k); kx3=-1*pi;
W=Wilson_loop(kx3,gamma_x,gamma_y,lambda,t,Ly); %求解Wilson loop
[nu_kx,E1]=eig(-1i*logm(W)); % wannier hamiltonian
[B,I]=sort(real(diag(E1)));
nu_j=sort((real(diag(E1)))/(2*pi)); % nu_j 按照能量实部排序
[V,~]=eig(W);
for i=1:length(I)
nu_kx1(:,i)=nu_kx(:,I(i)); % Wilson loop 的本征态
end
for i=1:length(kx1)
kx=kx1(i); H=[]; rho_j=zeros(Ly,1);
H=H_3(kx,gamma_x,gamma_y,lambda,t,Ly);
[V,~]=eig(H);
u_kx=V(:,1:end/2);
for j=1:N_occ*Ly
for n=1:N_occ*Ly
for ialpha=1:N_orb % sum alpha
for iy=1:Ly
rho_j(iy)=rho_j(iy)+abs(u_kx((iy-1)*N_orb+ialpha,n)...
*nu_kx1(n,j)).^2*nu_j(j);
end
end
end
end
rho_j1(:,i)=rho_j;
end
rho_j=sum(rho_j1,2);
rho=rho_j/N;
sum(rho(1:10))
Ry=[1:Ly];
plot(Ry,rho,'bo-');
xlabel('R_y');
ylabel('p_x');
function H_total=H_3(kx,gamma_x,gamma_y,lambda,t,Ly) %Ly 元胞数
H_0=[zeros(2) [lambda*exp(1i*kx)+gamma_x gamma_y;-gamma_y gamma_x+lambda*exp(-1i*kx)];...
[lambda*exp(-1i*kx)+gamma_x -gamma_y; gamma_y gamma_x+lambda*exp(1i*kx)] zeros(2)];
delta=1e-4; % delta=1e-4 引入微扰消除简并
H_0=H_0+eye(4)*2*t*cos(kx)+diag([-delta -delta delta delta]);
H_1=zeros(4);
H_1(1,4)=lambda; H_1(3,2)=-lambda;
H_1=H_1+eye(4)*t;
H_total=kron(eye(Ly),H_0)+kron(diag(ones(Ly-1,1),1),H_1)+kron(diag(ones(Ly-1,1),-1),H_1'); % ' .' 区别
end
% large Wilson loop
function W=Wilson_loop(kx,gamma_x,gamma_y,lambda,t,Ly)
vector=[]; num_k=51;
kx1=linspace(kx,kx+2*pi,num_k);
for i=1:length(kx1)
kx=kx1(i);
H=H_3(kx,gamma_x,gamma_y,lambda,t,Ly);
Size_W=size(H,1)/2;
[V,~]=eig(H);
if kx~=kx1(end) % kx+2*pi 多个威尔逊环
for ii=1:Size_W
vector(:,ii,i)=V(:,ii);
end
else
vector(:,:,i)=vector(:,:,1);
end
end
W1=eye(Size_W);
for i=1:length(kx1)-1
F=zeros(Size_W,Size_W,length(kx1));
for m=1:size(vector,2)
for n=1:size(vector,2)
F(m,n,i)=dot(vector(:,m,i+1),vector(:,n,i));
end
end
W1=F(:,:,i)*W1;
end
W=W1;
end
参考文献
不好意思,发重复了,第一次没有上传成功。参考文献是采参考资料[2]
哦哦,好的,我微信上回复你。
你好,请问可以利用这种方法计算高阶拓扑半金属中Weyl点的拓扑荷数吗,其思路是否类似?
看定义和公式是否一样了。如果只考虑某个动量截面的高阶拓扑不变量,计算应该是类似的。参考这篇文章以及其补充材料:Higher-Order Weyl Semimetals。
好的,谢谢您的回答。
关老师您好,我最近在研究拓扑绝缘体的极化时遇到一些问题,想请假一下您。就是对于某些二维模型,比如二维SSH模型,其边界态可以由对应方向的极化来解释,但是如果把边界取成正方形对角线方向,依然有局域的边界态,但沿着新的边界方向并没有之前的极化;再比如BBH四极矩模型,沿着对角线方向取边界也会有局域态,但沿着新边界(虽然我不确定能不能这么算)算nested Wilson loops对应的极化也消失了。但是模型bulk的性质并没发生改变。所以对于新边界上的边界态似乎没有对应的极化可以描述,是否有别的方法能解释这些特殊边界态呢?
我不确定你算的对不对。如果考虑斜对角,布里渊其还是原来的形状,所以在求nested Wilson loops的时候要注意k的范围。
嗯我知道布里渊区是之前的形状,但毕竟我想求对角线方向上的nested Wilson loops的极化,所以我把布里渊区分割拼接成一个斜的长方形(宽1/sqrt(2),长2/sqrt(2)),然后对角线方向就和长方形的长宽对应了,之后我再算nested Wilson loops的极化,就消失了,但边界态还在。您觉得我这样处理有问题吗
我觉得可能没什么问题,计算结果好像也是正常的,因为这样算的极化对应的“角态”在物理上就不是原来那个位置,所以没有量子化。拓扑平庸的边界态很常见,不代表什么。
好的,谢谢关老师
关老师,这个嵌套威尔逊环的代码解决了以前波函数的问题吗?
简并问题还没解决。目前暂时没做这方面的,之后如果解决了会更新。
作者您可以了解一下实空间中体四极矩qxy拓扑不变量,和嵌套威尔逊环等价,但某些情况下更鲁棒性
DOI: 10.1103/PhysRevLett.125.166801
DOI: 10.1103/PhysRevB.101.195309
嗯,感谢告知和提供的文献。我之前有大概了解一些,但事情比较多就暂且搁置了,之后有空再考虑考虑。这个链接有提供了相关的代码,但我还不确定是不是对的,供参考:https://github.com/kabume/Qc_real_space_method。
嗯嗯,非常感谢关老师
套其他玩具模型验证过了,GitHub代码的算体四极矩的思想是正确的
能否请教下代码里的求Qxy好像没有像文章公式里乘根号下对角酉矩阵Q。这是为什么呢
这个是实空间的算法吧
哪个文章?可以加我QQ:2437082289讨论
关老师,我想请教一下,算完wilson矩阵的本征后不是已经算是得出wannier了吗?为什么还要加入下面这段代码呢?
for i0 in range(2):
if np.real(nu_x[i0]) < 0:
nu_x[i0] += 1
nu_x = np.sort(nu_x)
nu_x_array.append(nu_x.real)
本征值有一个负值,由于在定义上只关心mod 1后的量,所以可以把负数改为正数。后面是对本征值进行排序,方便画图用的,不然连线会来回跳跃。
非常感谢!
这个能否有个Matlab程序
目前没有的。可以直接根据定义公式来写
你好,请问对于非四方布里渊区的体系,比如kagome晶格的六边形布里渊区,在计算Wilson loop的时候如何确定loop的方向?
看你要研究什么问题了,一般来说只要是闭合的曲线都能定义Wilson loop。这个我也不了解,还没怎么考虑,你可以查下文献,例如这两篇:
Hi, 我加你微信了 再次通过您的代码学习了很多
简并情况我尝试了一下 通过简并微扰论貌似可以区分这写简并态
结果我发您邮箱吧 有空我们可以讨论讨论
兄弟 你的简并问题解决了吗?可以加个联系方式吗?qq:2437082289
威尔逊环怎么看呢?或者怎么判断体系的拓扑性质呢?怎么预测角态边界态呢?
看下参考文献。计算对应的高阶拓扑不变量可以预测该拓扑性质,如果高阶拓扑不变量不为零,则存在量子化的角态。